Northwestern has a very collaborative environment which makes my cross-disciplinary research possible.”
Donna Hooshmand
PhD Candidate in Computer Science
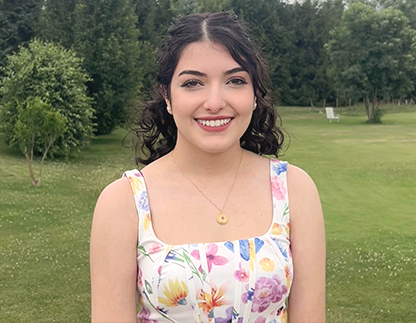
Donna Hooshmand is a PhD candidate in Computer Science in the McCormick School of Engineering. Her research bridges disciplines, applying artificial intelligence (AI) to real-world challenges in medicine, law, and data science. Donna was recognized by her program as one of the Winter 2023 Outstanding Teaching Assistant and Peer Mentors. She also works in the Cognition, Creativity, and Communication (C3) Lab, led by Professor Kristian Hammond.
How would you describe your research and/or work to a non-academic audience?
Broadly, I work on the applications of AI in order to lower the barrier to entry in using AI systems. My research is highly interdisciplinary and requires collaborations between experts in different fields.
We propose an Analytics-Augmented Generation (AAG) method that analyzes structured data to create sets of facts that guide generation. The AAG approach enables the use of standard analytic techniques to produce facts, which are then transformed into text and provided to an LLM. Our Solution, SATYRN, is a neurosymbolic platform that employs AAG to create accurate, fluent, and coherent reports from large-scale databases. Using AAG, SATYRN implements standard relational database analysis techniques to extract the desired information for a report, generating raw results in standardized language. Then, SATYRN calls a LLM to turn the raw results into a comprehensive report on the desired information. Separating the analysis and language generation processes steers LLMs using facts derived from relational databases which improves the overall accuracy of the final report.
Our findings show that SATYRN can generate fluent and coherent reports with factual accuracy of 86% or higher. SATYRN is domain agnostic and provides the ability to ask questions and generate a document from a relational database. However, it requires a domain map to function. This domain map is a lightweight knowledge representation that describes the objects in the database in order to apply analytics and produce information. TYTAN automates the domain mapping process, eliminating the technical barrier to entry that exists with SATYRN. Our goal is to increase accessibility to data analysis, by automating report generation from large data bases. TYTAN's interface with SATYRN helps achieve this goal by automating a task that normally would be performed by a skilled data scientist.
In addition to this work, I also work on applications of AI in medicine and have collaborated with Ophthalmologists at the Feinberg School of Medicine to use machine teaching, interactive machine learning, and curriculum learning as an alternative to traditional machine learning methods. Our goal in these projects is to pick the most optimal strategy and training set to minimize the required training data and the labels that doctors need to provide. We developed a model that identifies Retinal Ischemic Perivascular Lesions (RIPLs), an emerging ocular imaging biomarker of cardiovascular disease. Using only 495 training scans, our model achieved a sensitivity of 87%, and a specificity of 77% on a testing set of 50 Optical Coherence Tomography (OCT) scans. After the success of this project, we’ve expanded our work to detect Geographical Atrophy (GA) in OCT scans.
Tell us what inspired your research and/or work.
As our daily systems become more complex, the imbalance between the knowledge of non-technical users and the internal working structures of each system increases. This makes it difficult for end-users to feel comfortable using such systems, and furthermore, it makes it nearly impossible for them to modify systems to better fit their needs. In my research, I aim to bridge the gap for non-technical users to interact with complex systems and to make such systems widely accessible.
What do you find both rewarding and challenging about your research and/or work?
Throughout my program I’ve taken the lead on supervising undergraduate and master's students in research and mentoring them in their own academic journeys. This requires a unique curriculum for each student based on their initial knowledge, goals, and interests.
It can be a struggle to find a balance between direct mentorship and personalized assignments to drive the students to their goals. This generates a positive and productive environment that facilitates better research outcomes. It’s rewarding to see the students under my mentorship succeed, and even more rewarding when I can learn something from them as well.
What is the biggest potential impact or implication of your work?
Allowing non-technical users to easily access and train a system allows domain experts to transfer their knowledge easily. This will allow more specialized systems to be created efficiently by domain experts and allow more use of AI in different fields.
Why Northwestern?
Northwestern has a very collaborative environment which makes my cross-disciplinary research possible. The Computer Science + X (CS+X) initiative fosters interdisciplinary work between different schools and departments. Since my research is focused on applications of AI, these collaborations are crucial to finding real-world problems to solve. My research spans collaborations across computer science, medicine, law, and data science.
How do you unwind after a long day?
I watch TV series (in both Farsi and English) in a lot of different genres, play video games, and watch the Sea Otter live cam at the Vancouver Aquarium or the Monterey Bay Aquarium!
Publish Date: December 10, 2024
If you know a graduate student, postdoctoral scholar, graduate faculty member, staff member, or a member of our TGS alumni population who would make a great candidate for our TGS Spotlight Series, please complete this brief TGS Spotlight Series Nomination Form.